A new scientific analysis of nearly 4,000 mutations deliberately
engineered into the BRCA1 gene will immediately benefit people
undergoing genetic testing for breast or ovarian cancer risk.
The study was published today in the Sept. 12 edition of the
scientific journal, Nature. Additional data from the research has been
made available online. Please see the Nature paper
Jay Shendure and Lea Starita, faculty in the Department of Genome
Sciences at the University of Washington School of Medicine, are the
senior authors. Shendure directs the Brotman Baty Institute for
Precision Medicine in Seattle, which helped support the study. Starita
co-directs the Brotman Baty Advanced Technology Lab. Shendure is also a
Howard Hughes Medical Institute investigator.
Greg Findlay, an M.D./Ph.D. student in the physician-scientist
training program at the UW medical school, led the study, which is
entitled, “Accurate classification of BRCA1 variants with saturation
genome editing.”
Stephen Chanock of the National Cancer Institute wrote a Nature commentary on the study. It's also featured in Nature News, "Huge genetic-screening effort helps pinpoint roots of cancer."
The BRCA1 gene suppresses tumors, but the exact mechanisms by which
it does this are not fully understood. Certain mutations in the gene are
known to predispose women to breast and ovarian cancers. If a healthy
woman undergoes genetic testing and a cancer-predisposing mutation is
found, surgery or more frequent screening can greatly reduce her risk of
ever getting those types of cancer.
However, many women undergoing genetic screening for breast and
ovarian cancer learn that their BRCA1 gene contains a variant of
uncertain significance. This is a mutation that is not currently known
to cause cancer, but that theoretically could. BRCA1 is an exceptionally
well-studied gene, but at present thousands of mutations in it fall
into this category. Their implications for cancer risk are unknown.
“For example,” Shendure said, “I might sequence the BRCA1 gene in a
woman and observe that she has a mutation, but I don’t know whether that
mutation will actually lead to an increased risk of breast cancer or
whether it will be perfectly harmless.”
These variants of uncertain significance, the scientists wrote in
their report, fundamentally limit the clinical utility of a patient’s
genetic information. There is a concern, Findlay said, that women who
harbor variants that indeed lead to cancer are not identified during
genetic testing, and therefore are not presented with options that might
allow them to avoid breast cancer or detect it in its more treatable
stages.
The BRCA1 gene has been sequenced in millions of women in the United
States alone over the past decade. The idea that mutations in a gene
could account for some cases of breast cancer, as well as the mapping of
the BRCA1 gene’s location on chromosome 17, was proposed in 1990 by
geneticist and epidemiologist Mary-Claire King, after reviewing breast
cancer inheritance patterns in families. King joined the UW medical
school faculty in 1995.
“Frequently women are being tested for BRCA1 mutations because they
have a family history of breast or ovarian cancer,” Starita said. “To be
told that they have a genetic variant in this cancer predisposing gene,
but that the doctor doesn’t know what it means, does not reduce their
stress or their anxiety.”
“The challenge with BRCA1 testing, and with genetic testing more
generally, is even though the cost of genome sequencing has plummeted,
we continue to have trouble interpreting what that information means,”
Shendure said. “The premise of precision medicine that we’ve been
promising for 10 or 15 years now is that we’ll sequence the genomes of
ordinary citizens, and that information will improve their health
outcomes. We hope this new study brings us one step closer to delivering
on that promise.”
To help clinicians and patients obtain better information about
genetic variants, Findlay developed a research approach called
saturation genome editing. This method relies on CRISPR, an enzyme tool
that cuts strands of DNA to modify its sequence. With it, they made
thousands of miniscule revisions in the BRCA1 gene, even changes that
have not yet been seen in a human. Then the scientists measured the
effects of each mutation to see which ones caused problems in human
cells growing in a dish.
“Being able to break it down at the level of single base pairs of DNA
was pretty exciting,” the researchers said. “We found that you can
study changes in the BRCA1 gene in the lab, and they reflect with
surprising accuracy what happens in a person with that variant. Even
though we’re working with cells in a dish, when we put changes into the
genomes of those cells, and look at the places where the right answers
should be, they almost always line up with what we observe in patients.”
Previously only a few different mutations could be examined at a
time. Now, the means to edit a gene into myriad versions is making it
easier to understand how our DNA functions.
“We are basically categorizing all of the possible changes across
critical regions of the BRCA1 gene as either behaving like
disease-causing mutations or not,” Findlay said. The researchers needed
about six months to test almost 4,000 mutations. They are extending
this work to cover the entire BRCA1 gene over the next couple of years.
The researchers are quickly releasing newly available variant
information through the Brotman Baty database to assist patients and
clinicians trying to figure out what a test result might mean.
“Our hope,” Starita said, “is that this database will continue to
grow and will become a central point for guiding the interpretation of
actionable variants as they are first observed in women.”
“The ability to study in a dish what will happen in a living,
breathing human, at scale, opens up a lot of possibilities in medical
genetics,” Shendure added. The scientists plan to apply the saturation
genome editing methods to other cancer risk genes.
“The study serves as a blueprint,” Findlay noted, “for how to test
rare mutations in important genes that have big consequences for human
health.”
Monday, September 17, 2018
What Causes Breast Cancer? Causes of Breast Cancer Explained
One of the essential parts of treating disease is understanding the
etiology. Through the comprehension of disease many therapeutic targets
are developed. However, often we do not know what exactly causes disease
and only comprehend partially the pathogenesis of the disease. An
example of this is breast cancer.
Breast cancer is one of the most common types of cancer in women. Like other types of cancers, it is a heterogeneous disease than can be classified in several ways. The most common of these is through histological or molecular sub types. Even though in some particular cases the causes of breast cancer can correspond to a particular clinical sub type of cancer, often this is not the case. Additionally, in most cases of breast cancer, no specific cause can be identified.
Current understanding of the causes of breast cancer has
been the product of integrating the general mechanism by which all
cancer originates from with the specific causes of breast cancer. Therefore,
in order to understand what causes breast cancer, one must first
understand general process of carcinogenesis and the associated
characteristics resulting from it. After this, one must integrate the
currently known risk factors for breast cancer to this process.
Carcinogenesis is the process by which regular cells become malignant cells. This process is often multifactorial and of great complexity. This complexity arises from the numerous causes and interaction between these causes. In general, the causes of cancers are classified as exogenous, endogenous or genetic.
The exogenous causes of cancers are labeled as carcinogens due to their capacity to induce carcinogenesis in cells. The World Health Organization classifies carcinogens according to their nature into three groups, chemical, physical and biological. Even though there is strong epidemiological about almost all known carcinogen, the mechanism by which they produce cancer is unknown.
Chemicals, like the other two groups, are a varied group of carcinogens originating from diverse sources and with great differences in their structure. Chemical carcinogens include both inorganic compounds (such as nickel, cadmium, and arsenic) and organic compounds (such as nitrosamines or trichloroethylene). The mechanisms by which they produce cellular lesion are incredibly diverse. Additionally, a single chemical compound can produce lesion through several disruptions. Hormones and hormone-like compounds are known chemical causes of breast cancer. Interacting directly with some breast cancer through estrogen receptors or through the stimulation of inflammatory pathways. Recently, evidence for the role of estrogen receptors has been found in the increased risk of breast cancer in patients with hormonal therapy.
Another group of carcinogens includes the physical agents. Physical forces can also have a tremendous effect on cells, which can later result in cancer. Among the physical carcinogens are ultraviolet radiation, iatrogenic sources of radiation and radiation from materials such as uranium. Physical agents are also candidates to participating the multifactorial cause of breast cancer. Specifically, iatrogenic radiation has been linked to increased risk of breast cancer.
The last group of exogenous carcinogens is the biological group. These include several viruses and bacteria that act as carcinogens in mankind. Among these are the human papilloma virus (HPV16 and HPV18), human herpes virus 8, the Epstein-Barr virus and Helicobacter pylori. Biological causes are associated with numerous cancers such as cervical cancer, Kaposi sarcoma, lymphomas and gastric cancer. But, their role in breast cancer has not been documented.
Carcinogens are not only exogenous but can also emerge from a process within the body. These carcinogens are labeled, in contrast, endogenous. However, whether endogenous carcinogens are considered true causes of cancer is subject to debate because they act as mediators of exogenous carcinogens. Nevertheless, their role in the development of cancer is essential. Among the endogenous carcinogens are the reactive oxygen species and inflammatory mediators. These trigger numerous responses in the body that can lead to cancer development. For example, breast cancer has been linked to many diseases characterized by an increased inflammatory state such as obesity or type 2 diabetes.
Often, in cancer, the exogenous and endogenous carcinogens interact with the genetic makeup of patients. The process by which genes interact has been well described in scientific literature. Cancer is essentially a genetic disease, but not in the traditional description. Cancer cells are characterized by damage to its genetic material but most of this damage is not the product of mutation in the germline. Cancer, in contrast, is the result of somatic mutations which accumulate in certain cells. This can be observed in cancer where 80 to 90% of cases of breast cancer are not explained by hereditary factors. This does not exclude the effect of inherited mutations that can lead to the remaining 10 to 20% of cases of breast cancer. Regardless of the predominance of environmental causes, inherited mutations need to be considered.
The mutations, either somatic or inherited, associated with the development of cancer are restricted to a few particular types of genes. These are labeled as cancer genes and include tumor suppressor genes, oncogenes, DNA repair and checkpoint genes, risk modulating genes and execution genes.
As a result of the complex interaction between the carcinogens and the genes associated with the cancerous process, cancer cells are created. These cells will have some characteristics and behavior which allow it to grow and later metastasize. These characteristics were summarized in the year 2000 by Hanahan and Weinberg as the Hallmarks of Cancer. The six classical hallmarks are resisting cell death, sustaining proliferative signaling, evading growth suppressors, inducing angiogenesis, enabling replicative immortality and activating invasion and metastasis. As a result of recent discoveries additional hallmarks are currently being proposed, such as the evasion of immune destruction and the dysregulation of cellular energetics. All of these, also have a role in explaining how to do the interactions between the genetic alterations and carcinogens cause breast cancer.
Most of the previous causes and process are can be observed as the specific causes of breast cancer. These, like for other cancers, can be classified into genetic and environmental causes.
The genetic causes of breast causes include avarious mutation in genes which result in different increases in the patient´s risk of breast cancer. An inverse relationship with the risk of cancer to the frequency of the mutation. Due to these differences genes can be grouped as common (low risk) genes, rare (moderate risk) genes, and very rare (high risk) genes.
The group of the high-risk genetic causes of breast cancer is one of the most researched and known mutations involved in breast cancer. The most common and representative mutations occur in the BRCA1 and BRCA2. The name of these genes originates from their role in breast cancer (BR + CA). These high-risk mutations are including some of the known hereditary breast cancers. But, in sporadic cases of breast cancer mutations in these genes are also observed. The BRCA1 and BRCA2 are examples of tumor suppressor genes involved in DNA repair and control of homologous recombination. As result, BRCA1 and BRCA2 mutations are associated with genetic instability and dysregulation of cell replication which can cause breast cancer.
The protein coded by the BRCA2 gene, interacts, and control numerous proteins involved in DNA repairs. These proteins are coded by genes which can cause breast cancer if mutated. These include the ATM gene, CHK genes, PALB2 gene and among others.
Germline mutations in BRCA1 and BRCA2are associated with 40-80% lifetime risk of breast cancer. This a significant increase in risk when compared to the general population lifetime risk of breast cancer, 10%. Mutation of other genes is also associated with an increased lifetime risk of breast cancer. These include 4 breast cancer genetic syndromes, Li-Fraumeni Syndrome (P53), Cowden Syndrome (PTEN), Hereditary Diffuse Gastric Syndrome (CDH1) and Peutz-Jeghers Syndrome (STK11). As a syndrome, apart from an increased risk of breast cancer, they have other associated features, which include another type of cancers or precancerous changes.
One of the syndromes associated with hereditary causes of breast cancers is the Li-Fraumeni syndrome. Produced by the mutation of a tumor suppressor gene, TP53,a patient with this syndrome has a risk of breast cancer ranging between 50-80% by the age of 45. This gene codes for a protein that regulates gene stability in general tissues, resulting in additional risk of other types of cancers such as acute leukemia, lymphomas and brain tumors.
Another tumor suppressor gene associated with hereditary causes of breast cancer is the CDH1 gene. Mutations in this gene cause Hereditary Diffuse Gastric Syndrome by causing dysfunction of DNA mismatch repair, causing genomic instability. This results in a 39% lifetime risk of breast cancer, specifically lobar cancer. Additionally, like seen in its name, it can also cause gastric cancers.
STK 11 is another gene associated with a breast cancer genetic syndrome, the Peutz-Jeghers syndrome. Like the previous causes of hereditary breast cancer, the STK 11 gene codes for a tumor suppressor gene. However, its mutation affects the regulation of apoptosis (resulting in evasion of apoptosis) and the use of energy. The Peutz-Jeghers syndrome represents a risk of breast cancer of 32% by the age of 60. Like the others, this syndrome also manifests as an increase in the risk developing other cancers such as cervical adenoma malignum or lung cancer.
The Cowden Syndrome, in contrast to the previous hereditary breast cancer syndromes, is not the result of alterations of a tumor suppressor gene. Rather, it’s affected gene PTEN codes for an enzyme of the PI3K pathway. Nevertheless, it also raises the risk of breast cancer and other conditions.
The moderate risk genetic causes of breast cancer are a group composed of several genes which code for a protein involved in BRCA1 and BRCA2 functions. Some, such as the CHK genes increase the lifetime risk of breast cancer to a 20-80%. Others, like the ATM gene and the PALB2 gene, increase the cancer risk in a twofold. It is important to recall that even though mutations in these genes carry a lower risk, they occur more often. Perhaps this due to the dependency of the interaction with environmental causes of breast cancers. This also occurs with the low-risk genes which include the TOX3 gene, the MAP3K1 gene, theLSP1gene, and the CASP8.
Provided that few cases are the result of hereditary causes of breast cancer and that mutation only accounts for some cases, the influence of the environment is evident in the development of breast cancer. The environmental causes of breast cancer include the exposition of patients to ionizing radiation, exposure to estrogens and a high-fat diet.
The effects of ionizing radiations and its association with cancer are evident. Through the interaction with genes, this physical cause of breast cancer can provoke genomic instability in patients. Studies have shown an increased risk of breast cancer treated with ionizing radiation associated with ATM gene mutations or independent to this gene. Additionally, it has been pointed out that excessive screening through frequent mammography can, ironically, increase the risk in patients.
Estrogen exposure has also been identified as an important risk factor in the development of breast cancer. This chemical cause of breast cancer is associated with numerous aspect of female life such as an early menarche, late menopause, and fewer pregnancies. All of these can result in greater activation of receptors which stimulate proliferation pathways. Additionally, chemicals from industry, estrogen therapy or natural sources like soy have also been associated with higher risk of breast cancer. These effects are mediated through the estrogen receptors, thestructure responsible for the regulation of growth in the normal breast. More than 70% of breast cancer display the estrogen receptor, normally along with progesterone receptor. Estrogens additionally, through it metabolism, can produce a compound which produces reactive oxygen species. Which can either damage DNA or alter the energetically the general physiologic process in the cells?
Another environmental cause of breast cancer is a high-fat diet, a factor which interacts with many other causes. A patient with a high-fatdiet, first of all, can precipitate the development of type 2 diabetes, obesity, and dyslipidemias, which are all associated with an increased in the body inflammatory mediators and reactive oxygen species. Additionally, both the direct high-fat diet and the obesity that can follow have been observed to have an estrogenic effect.
The development of cancer through the linear multi step model can organize the causes of breast cancer in a logical manner. Initially, breast tissue is found devoid of histological changes. The only alterations could be inherited gene mutations which predispose to the disease but these require the input from environmental factors. Some of the initial environmental factors are the exposition to estrogen receptor stimulators and as consequence abnormal response in these receptors develops Additionally, as a consequence of this chemical cause of breast cancer, these cells begin to avoid apoptotic signals. Soon after this, tumor-suppressor dysfunction develops causing genetic instability and the expression of abnormal oncogenes. As a consequence, cells become cancerous cells in the breast and can behave as an in situ or invasive cancers.
This model associates both environmental and inherited causes of breast cancer to the accumulation of genetic alterations. These, in turn, can start a snowball effect of causing further instability, resulting in more genetic alterations. This can provide a logical understanding of what process and factors cause breast cancer, which allows the current physician to target some of these as pharmacological targets. Nevertheless, due to the complex nature of the causes of breast cancer, much more studies are needed to understand the diverse interaction among the causes. This way is allowing scientists and physicians to provide better treatment and prognosis to patients.
Breast cancer is one of the most common types of cancer in women. Like other types of cancers, it is a heterogeneous disease than can be classified in several ways. The most common of these is through histological or molecular sub types. Even though in some particular cases the causes of breast cancer can correspond to a particular clinical sub type of cancer, often this is not the case. Additionally, in most cases of breast cancer, no specific cause can be identified.
What Causes Breast Cancer?
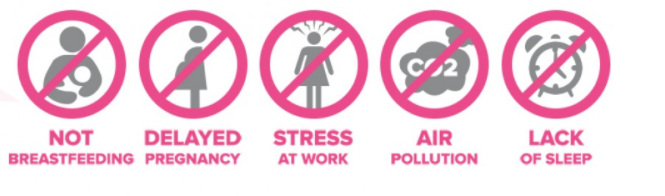
Breast Cancer Causes
Carcinogenesis is the process by which regular cells become malignant cells. This process is often multifactorial and of great complexity. This complexity arises from the numerous causes and interaction between these causes. In general, the causes of cancers are classified as exogenous, endogenous or genetic.
The exogenous causes of cancers are labeled as carcinogens due to their capacity to induce carcinogenesis in cells. The World Health Organization classifies carcinogens according to their nature into three groups, chemical, physical and biological. Even though there is strong epidemiological about almost all known carcinogen, the mechanism by which they produce cancer is unknown.
Chemicals, like the other two groups, are a varied group of carcinogens originating from diverse sources and with great differences in their structure. Chemical carcinogens include both inorganic compounds (such as nickel, cadmium, and arsenic) and organic compounds (such as nitrosamines or trichloroethylene). The mechanisms by which they produce cellular lesion are incredibly diverse. Additionally, a single chemical compound can produce lesion through several disruptions. Hormones and hormone-like compounds are known chemical causes of breast cancer. Interacting directly with some breast cancer through estrogen receptors or through the stimulation of inflammatory pathways. Recently, evidence for the role of estrogen receptors has been found in the increased risk of breast cancer in patients with hormonal therapy.
Another group of carcinogens includes the physical agents. Physical forces can also have a tremendous effect on cells, which can later result in cancer. Among the physical carcinogens are ultraviolet radiation, iatrogenic sources of radiation and radiation from materials such as uranium. Physical agents are also candidates to participating the multifactorial cause of breast cancer. Specifically, iatrogenic radiation has been linked to increased risk of breast cancer.
The last group of exogenous carcinogens is the biological group. These include several viruses and bacteria that act as carcinogens in mankind. Among these are the human papilloma virus (HPV16 and HPV18), human herpes virus 8, the Epstein-Barr virus and Helicobacter pylori. Biological causes are associated with numerous cancers such as cervical cancer, Kaposi sarcoma, lymphomas and gastric cancer. But, their role in breast cancer has not been documented.
Carcinogens are not only exogenous but can also emerge from a process within the body. These carcinogens are labeled, in contrast, endogenous. However, whether endogenous carcinogens are considered true causes of cancer is subject to debate because they act as mediators of exogenous carcinogens. Nevertheless, their role in the development of cancer is essential. Among the endogenous carcinogens are the reactive oxygen species and inflammatory mediators. These trigger numerous responses in the body that can lead to cancer development. For example, breast cancer has been linked to many diseases characterized by an increased inflammatory state such as obesity or type 2 diabetes.
Often, in cancer, the exogenous and endogenous carcinogens interact with the genetic makeup of patients. The process by which genes interact has been well described in scientific literature. Cancer is essentially a genetic disease, but not in the traditional description. Cancer cells are characterized by damage to its genetic material but most of this damage is not the product of mutation in the germline. Cancer, in contrast, is the result of somatic mutations which accumulate in certain cells. This can be observed in cancer where 80 to 90% of cases of breast cancer are not explained by hereditary factors. This does not exclude the effect of inherited mutations that can lead to the remaining 10 to 20% of cases of breast cancer. Regardless of the predominance of environmental causes, inherited mutations need to be considered.
The mutations, either somatic or inherited, associated with the development of cancer are restricted to a few particular types of genes. These are labeled as cancer genes and include tumor suppressor genes, oncogenes, DNA repair and checkpoint genes, risk modulating genes and execution genes.
As a result of the complex interaction between the carcinogens and the genes associated with the cancerous process, cancer cells are created. These cells will have some characteristics and behavior which allow it to grow and later metastasize. These characteristics were summarized in the year 2000 by Hanahan and Weinberg as the Hallmarks of Cancer. The six classical hallmarks are resisting cell death, sustaining proliferative signaling, evading growth suppressors, inducing angiogenesis, enabling replicative immortality and activating invasion and metastasis. As a result of recent discoveries additional hallmarks are currently being proposed, such as the evasion of immune destruction and the dysregulation of cellular energetics. All of these, also have a role in explaining how to do the interactions between the genetic alterations and carcinogens cause breast cancer.
Most of the previous causes and process are can be observed as the specific causes of breast cancer. These, like for other cancers, can be classified into genetic and environmental causes.
The genetic causes of breast causes include avarious mutation in genes which result in different increases in the patient´s risk of breast cancer. An inverse relationship with the risk of cancer to the frequency of the mutation. Due to these differences genes can be grouped as common (low risk) genes, rare (moderate risk) genes, and very rare (high risk) genes.
The group of the high-risk genetic causes of breast cancer is one of the most researched and known mutations involved in breast cancer. The most common and representative mutations occur in the BRCA1 and BRCA2. The name of these genes originates from their role in breast cancer (BR + CA). These high-risk mutations are including some of the known hereditary breast cancers. But, in sporadic cases of breast cancer mutations in these genes are also observed. The BRCA1 and BRCA2 are examples of tumor suppressor genes involved in DNA repair and control of homologous recombination. As result, BRCA1 and BRCA2 mutations are associated with genetic instability and dysregulation of cell replication which can cause breast cancer.
The protein coded by the BRCA2 gene, interacts, and control numerous proteins involved in DNA repairs. These proteins are coded by genes which can cause breast cancer if mutated. These include the ATM gene, CHK genes, PALB2 gene and among others.
Germline mutations in BRCA1 and BRCA2are associated with 40-80% lifetime risk of breast cancer. This a significant increase in risk when compared to the general population lifetime risk of breast cancer, 10%. Mutation of other genes is also associated with an increased lifetime risk of breast cancer. These include 4 breast cancer genetic syndromes, Li-Fraumeni Syndrome (P53), Cowden Syndrome (PTEN), Hereditary Diffuse Gastric Syndrome (CDH1) and Peutz-Jeghers Syndrome (STK11). As a syndrome, apart from an increased risk of breast cancer, they have other associated features, which include another type of cancers or precancerous changes.
One of the syndromes associated with hereditary causes of breast cancers is the Li-Fraumeni syndrome. Produced by the mutation of a tumor suppressor gene, TP53,a patient with this syndrome has a risk of breast cancer ranging between 50-80% by the age of 45. This gene codes for a protein that regulates gene stability in general tissues, resulting in additional risk of other types of cancers such as acute leukemia, lymphomas and brain tumors.
Another tumor suppressor gene associated with hereditary causes of breast cancer is the CDH1 gene. Mutations in this gene cause Hereditary Diffuse Gastric Syndrome by causing dysfunction of DNA mismatch repair, causing genomic instability. This results in a 39% lifetime risk of breast cancer, specifically lobar cancer. Additionally, like seen in its name, it can also cause gastric cancers.
STK 11 is another gene associated with a breast cancer genetic syndrome, the Peutz-Jeghers syndrome. Like the previous causes of hereditary breast cancer, the STK 11 gene codes for a tumor suppressor gene. However, its mutation affects the regulation of apoptosis (resulting in evasion of apoptosis) and the use of energy. The Peutz-Jeghers syndrome represents a risk of breast cancer of 32% by the age of 60. Like the others, this syndrome also manifests as an increase in the risk developing other cancers such as cervical adenoma malignum or lung cancer.
The Cowden Syndrome, in contrast to the previous hereditary breast cancer syndromes, is not the result of alterations of a tumor suppressor gene. Rather, it’s affected gene PTEN codes for an enzyme of the PI3K pathway. Nevertheless, it also raises the risk of breast cancer and other conditions.
The moderate risk genetic causes of breast cancer are a group composed of several genes which code for a protein involved in BRCA1 and BRCA2 functions. Some, such as the CHK genes increase the lifetime risk of breast cancer to a 20-80%. Others, like the ATM gene and the PALB2 gene, increase the cancer risk in a twofold. It is important to recall that even though mutations in these genes carry a lower risk, they occur more often. Perhaps this due to the dependency of the interaction with environmental causes of breast cancers. This also occurs with the low-risk genes which include the TOX3 gene, the MAP3K1 gene, theLSP1gene, and the CASP8.
Provided that few cases are the result of hereditary causes of breast cancer and that mutation only accounts for some cases, the influence of the environment is evident in the development of breast cancer. The environmental causes of breast cancer include the exposition of patients to ionizing radiation, exposure to estrogens and a high-fat diet.
The effects of ionizing radiations and its association with cancer are evident. Through the interaction with genes, this physical cause of breast cancer can provoke genomic instability in patients. Studies have shown an increased risk of breast cancer treated with ionizing radiation associated with ATM gene mutations or independent to this gene. Additionally, it has been pointed out that excessive screening through frequent mammography can, ironically, increase the risk in patients.
Estrogen exposure has also been identified as an important risk factor in the development of breast cancer. This chemical cause of breast cancer is associated with numerous aspect of female life such as an early menarche, late menopause, and fewer pregnancies. All of these can result in greater activation of receptors which stimulate proliferation pathways. Additionally, chemicals from industry, estrogen therapy or natural sources like soy have also been associated with higher risk of breast cancer. These effects are mediated through the estrogen receptors, thestructure responsible for the regulation of growth in the normal breast. More than 70% of breast cancer display the estrogen receptor, normally along with progesterone receptor. Estrogens additionally, through it metabolism, can produce a compound which produces reactive oxygen species. Which can either damage DNA or alter the energetically the general physiologic process in the cells?
Another environmental cause of breast cancer is a high-fat diet, a factor which interacts with many other causes. A patient with a high-fatdiet, first of all, can precipitate the development of type 2 diabetes, obesity, and dyslipidemias, which are all associated with an increased in the body inflammatory mediators and reactive oxygen species. Additionally, both the direct high-fat diet and the obesity that can follow have been observed to have an estrogenic effect.
The development of cancer through the linear multi step model can organize the causes of breast cancer in a logical manner. Initially, breast tissue is found devoid of histological changes. The only alterations could be inherited gene mutations which predispose to the disease but these require the input from environmental factors. Some of the initial environmental factors are the exposition to estrogen receptor stimulators and as consequence abnormal response in these receptors develops Additionally, as a consequence of this chemical cause of breast cancer, these cells begin to avoid apoptotic signals. Soon after this, tumor-suppressor dysfunction develops causing genetic instability and the expression of abnormal oncogenes. As a consequence, cells become cancerous cells in the breast and can behave as an in situ or invasive cancers.
This model associates both environmental and inherited causes of breast cancer to the accumulation of genetic alterations. These, in turn, can start a snowball effect of causing further instability, resulting in more genetic alterations. This can provide a logical understanding of what process and factors cause breast cancer, which allows the current physician to target some of these as pharmacological targets. Nevertheless, due to the complex nature of the causes of breast cancer, much more studies are needed to understand the diverse interaction among the causes. This way is allowing scientists and physicians to provide better treatment and prognosis to patients.
What's your breast cancer risk? This study aims to tell you precisely
Breast cancer is one of the most worrisome diagnoses a woman can get, and now women know that they can get a heads-up if they have an extra-high risk by getting a genetic test.
Mutations in dozens of different genes can raise the risk of breast cancer, but the two best-known risk genes are BRCA1 and BRCA2. Everyone carries these genes. When breast cancer, ovarian cancer or prostate cancer runs in a family, doctors often advise getting a test to assess whether a patient carries a risky version of one of them.
Now there are home tests for common BRCA1 and BRCA2 mutations. Some clearly raise a woman's risk of cancer, and she can get more regular mammograms or, as actress Angelie Jolie opted to do, have her breasts surgically removed.
However, many people have mutations called variants of uncertain significance. It’s not known whether they raise cancer risk.
Mutations are mistakes in the genetic code, represented by the letters A, T, C and G repeated over and over again. Big mistakes in the code mean that the BRCA genes don’t work right and don’t fix the damage in cells that can lead to cancer.
But the BRCA1 gene is 10,000 letters long. A lot of little mistakes can turn up in a sequence that long, and not all of them mean cancer.
How can you tell which ones do?
Jay Shendure at the University of Washington and colleagues think they’ve come up with a way to tell. They painstakingly created mutations one by one in a batch of cancer cells that die when they carry mutations that affect a gene’s function.
To do this, they used a precision DNA editing method called CRISPR. They created nearly 4,000 single-letter mutations, one by one, in their dishes of cells and found that they could predict which mutations might raise the risk of cancer.
They won’t be able to say precisely how those mistakes would affect development of cancer in a living human being, but when they compared their findings with known cancer-causing mutations, they aligned.
The method might be used to help tell people with so-called variants of uncertain significance whether they should worry.
“We predict that these results will be immediately useful for the clinical interpretation of BRCA1 variants,” they wrote in their report, published Wednesday in the journal Nature.
Breast cancer is the second-biggest cancer killer of American women, after lung cancer. The American Cancer Society says that every year, it's diagnosed in 260,000 women and a few men, and kills around 40,000.
About 12 percent of all women will develop breast cancer during their lives.
BRCA mutations are among dozens of other genetic mutations that raise the risk of breast or ovarian cancer. BRCA1 and BRCA2 are DNA repair genes, which find and fix cancer-causing mistakes elsewhere in the DNA code. When they carry mistakes themselves, the repair isn’t made, or it’s made improperly.
About 55 percent to 65 percent of women with a known cancer-causing BRCA1 mutation will develop breast cancer by age 70, the National Cancer Institute says. About 45 percent of women with faulty BRCA2 genes will.
This new approach might add to the list of risky mutations, and it may also eventually be used to predict the effects of other genetic mutations, the researchers said.
"If such a variant were present in a family member of mine, would I use this information? Absolutely," Shendure said in a statement.
Germline variation in ADAMTSL1 is associated with prognosis following breast cancer treatment in young women
To
identify genetic variants associated with breast cancer prognosis we
conduct a meta-analysis of overall survival (OS) and disease-free
survival (DFS) in 6042 patients from four cohorts. In young women,
breast cancer is characterized by a higher incidence of adverse
pathological features, unique gene expression profiles and worse
survival, which may relate to germline variation. To explore this
hypothesis, we also perform survival analysis in 2315 patients aged ≤ 40
years at diagnosis. Here, we identify two SNPs associated with
early-onset DFS, rs715212 (P
meta = 3.54 × 10−5) and rs10963755 (P
meta = 3.91 × 10−4) in ADAMTSL1.
The effect of these SNPs is independent of classical prognostic factors
and there is no heterogeneity between cohorts. Most importantly, the
association with rs715212 is noteworthy (FPRP <0.2) and approaches
genome-wide significance in multivariable analysis (P
multivariable = 5.37 × 10−8). Expression quantitative trait analysis provides tentative evidence that rs715212 may influence AREG expression (P
eQTL = 0.035), although further functional studies are needed to confirm this association and determine a mechanism.
Introduction
Breast
cancer is the second leading cause of cancer-related death in women
with nearly 450,000 deaths per year worldwide, despite advances in
effective chemotherapy1. Modern chemotherapy regimens include anthracyclines and increasingly taxanes, before and/or after surgery (http://www.cancerresearchuk.org/).
Patients are stratified according to clinical and pathological
characteristics of the cancer to predict prognosis, select treatment
regimen and to determine appropriate surgical options for individual
patients. Frequently, these decisions are made on the basis of
prognostic tools and guidelines which consider tumour characteristics
(size, grade and hormone receptors), nodal involvement, onset age,
family history and the mutation status of high-risk genes such as BRCA1 and BRCA2
2.
These prognostic tools help to balance the benefits of therapy against
their side effects. However, patients with the same tumour
characteristics and treatment frequently have different outcomes which
suggests that additional factors such as inherited variation may account
for these differences.
Many studies have demonstrated that germline variants contribute to the aetiology of breast cancer. They include several genome-wide association studies (GWASs) which have identified nearly 100 common low-penetrance breast cancer-associated alleles (odds ratios: 1.05–1.57)3. These low-penetrance alleles account for ~14% of the familial risk of disease while high-penetrance mutations in genes such as BRCA1 and BRCA2 and moderate-penetrance alleles in genes such as PALB2, ATM and CHEK2 account for a further ~16% of familial risk4.
Familial studies were among the first to indicate that inherited variants also influence breast cancer prognosis5 and many germline variants associated with survival have been identified. For example, germline mutations in CHEK2 6 and PALB2 7 have been implicated in poor prognosis and single-nucleotide polymorphisms (SNPs) have been associated with the risk of developing either oestrogen receptor (ER)-positive8 or -negative9 breast cancer subtypes, which have differing outcomes. More recently, many studies including GWASs have identified SNPs directly associated with breast cancer survival that are largely independent of traditional tumour prognostic factors10. Most of these loci have small effect sizes (hazard ratio (HR) < 1.5) and it is important to note that many loci reported by GWASs do not reach genome-wide significance which suggests that some of the previous GWASs were underpowered due to small sample size. Indeed, it has been suggested that extremely large sample sizes are needed to establish genome-wide levels of significance in breast cancer survival studies10. Alternatively, focussing on a smaller cohort of patients with a particular breast cancer subtype may increase power by reducing genetic heterogeneity. These genetic determinants of prognosis are important because they could improve prognostic models, aid selection of appropriate treatments, and suggest targets for new therapies. For example, tumour gene expression profiles perform equally well or better than clinicopathologic models, possibly because they reflect a larger component of germline determinants of gene expression in an established tumour than models based on clinicopathologic features11.
The principal aim of this study is to identify genetic determinants of breast cancer prognosis using a meta-analysis of four GWASs and a fifth replication cohort. We also investigate the role of common germline variation in a subset of patients with early onset (aged ≤ 40 years at diagnosis). Although breast cancer is uncommon in young women, with only 7% of patients aged ≤ 40 years at diagnosis and 1% < 30 years12, it represents the most frequent form of non-skin cancer in young women, accounting for ~40% of cases13. Furthermore, women diagnosed between the ages of 15 and 39 years have a lower 5-year survival rate (83.5%) than women aged 40 to 49 years (89.1%) (www.cancerresearchuk.org). The worse survival of early-onset cases has been attributed to a higher incidence of adverse pathological features (higher histological grade14, more frequent ER- and progesterone receptor (PR)-negative tumours)15,16 but multivariable analysis has demonstrated that age is an independent risk factor after adjusting for stage, treatment and tumour characteristics12. Analyses of gene expression profiles have shown that tumours arising in young women can be distinguished by 367 biologically relevant gene sets16 and that ER-positive tumours in premenopausal women overexpress AREG, TFPI2, AMPH, DBX2, RP5–1054A22.3 and KLK5, and underexpress ESR1, CYP4Z1, RANBP3L, FOXD2 and PEX3 17,18. The impact of epidemiological risk factors, such as obesity, also differs between premenopausal women, where it reduces the risk of ER-positive tumours, and postmenopausal women where it increases overall susceptibility19,20. These observations have led to the suggestion that, from an aetiological perspective, early-onset breast cancer may represent a different type of disease with a unique underlying biology and response to epidemiological risk factors that could be influenced by germline variation.
We identify three association signals with suggestive significance levels and without heterogeneity between cohorts (1: rs715212 and rs10963755; 2: rs12302097; and 3: rs410155). The most significant of these is rs715212, situated in intron 19 of ADAMTSL1, which increases the risk of disease progression but only in patients with early onset (aged ≤ 40 years at diagnosis). When adjusting for the known prognostic factors, this association approaches a genome-wide level of significance and is assessed as noteworthy by the false positive report probability (FPRP < 0.2). We also demonstrate that rs715212 is nominally associated with the expression of AREG. We therefore conclude that the association between ADAMTSL1 and breast cancer prognosis may involve an interaction with AREG expression, although further functional studies are needed to confirm this association and to determine the mechanism.
Many studies have demonstrated that germline variants contribute to the aetiology of breast cancer. They include several genome-wide association studies (GWASs) which have identified nearly 100 common low-penetrance breast cancer-associated alleles (odds ratios: 1.05–1.57)3. These low-penetrance alleles account for ~14% of the familial risk of disease while high-penetrance mutations in genes such as BRCA1 and BRCA2 and moderate-penetrance alleles in genes such as PALB2, ATM and CHEK2 account for a further ~16% of familial risk4.
Familial studies were among the first to indicate that inherited variants also influence breast cancer prognosis5 and many germline variants associated with survival have been identified. For example, germline mutations in CHEK2 6 and PALB2 7 have been implicated in poor prognosis and single-nucleotide polymorphisms (SNPs) have been associated with the risk of developing either oestrogen receptor (ER)-positive8 or -negative9 breast cancer subtypes, which have differing outcomes. More recently, many studies including GWASs have identified SNPs directly associated with breast cancer survival that are largely independent of traditional tumour prognostic factors10. Most of these loci have small effect sizes (hazard ratio (HR) < 1.5) and it is important to note that many loci reported by GWASs do not reach genome-wide significance which suggests that some of the previous GWASs were underpowered due to small sample size. Indeed, it has been suggested that extremely large sample sizes are needed to establish genome-wide levels of significance in breast cancer survival studies10. Alternatively, focussing on a smaller cohort of patients with a particular breast cancer subtype may increase power by reducing genetic heterogeneity. These genetic determinants of prognosis are important because they could improve prognostic models, aid selection of appropriate treatments, and suggest targets for new therapies. For example, tumour gene expression profiles perform equally well or better than clinicopathologic models, possibly because they reflect a larger component of germline determinants of gene expression in an established tumour than models based on clinicopathologic features11.
The principal aim of this study is to identify genetic determinants of breast cancer prognosis using a meta-analysis of four GWASs and a fifth replication cohort. We also investigate the role of common germline variation in a subset of patients with early onset (aged ≤ 40 years at diagnosis). Although breast cancer is uncommon in young women, with only 7% of patients aged ≤ 40 years at diagnosis and 1% < 30 years12, it represents the most frequent form of non-skin cancer in young women, accounting for ~40% of cases13. Furthermore, women diagnosed between the ages of 15 and 39 years have a lower 5-year survival rate (83.5%) than women aged 40 to 49 years (89.1%) (www.cancerresearchuk.org). The worse survival of early-onset cases has been attributed to a higher incidence of adverse pathological features (higher histological grade14, more frequent ER- and progesterone receptor (PR)-negative tumours)15,16 but multivariable analysis has demonstrated that age is an independent risk factor after adjusting for stage, treatment and tumour characteristics12. Analyses of gene expression profiles have shown that tumours arising in young women can be distinguished by 367 biologically relevant gene sets16 and that ER-positive tumours in premenopausal women overexpress AREG, TFPI2, AMPH, DBX2, RP5–1054A22.3 and KLK5, and underexpress ESR1, CYP4Z1, RANBP3L, FOXD2 and PEX3 17,18. The impact of epidemiological risk factors, such as obesity, also differs between premenopausal women, where it reduces the risk of ER-positive tumours, and postmenopausal women where it increases overall susceptibility19,20. These observations have led to the suggestion that, from an aetiological perspective, early-onset breast cancer may represent a different type of disease with a unique underlying biology and response to epidemiological risk factors that could be influenced by germline variation.
We identify three association signals with suggestive significance levels and without heterogeneity between cohorts (1: rs715212 and rs10963755; 2: rs12302097; and 3: rs410155). The most significant of these is rs715212, situated in intron 19 of ADAMTSL1, which increases the risk of disease progression but only in patients with early onset (aged ≤ 40 years at diagnosis). When adjusting for the known prognostic factors, this association approaches a genome-wide level of significance and is assessed as noteworthy by the false positive report probability (FPRP < 0.2). We also demonstrate that rs715212 is nominally associated with the expression of AREG. We therefore conclude that the association between ADAMTSL1 and breast cancer prognosis may involve an interaction with AREG expression, although further functional studies are needed to confirm this association and to determine the mechanism.
Results
Comparison of clinicopathologic features between cohorts
Stage-1 breast cancer samples came from four cohorts from Australia (Australian Breast Cancer Family Study (ABCFS)), Helsinki (Helsinki breast cancer study (HEBCS)), the United Kingdom (Prospective Study of Outcomes in Sporadic vs. Hereditary breast cancer (POSH)) and Germany (SUCCESS-A). A further 1303 independent patients from the POSH cohort were used for replication analysis at stage-2 (Table 1, see Methods for a full description of these cohorts). The baseline characteristics among the stage-1 and validation cohorts were significantly different (Table 1). These differences are largely due to the POSH cohort, which only recruited patients with early onset (age ≤ 40 years at diagnosis), and the selection of patients with triple-negative breast cancer (TNBC) or survival extremes from the POSH cohort at stage-1. As a result, the stage-1 POSH cohort had the highest frequency of ER, PR and HER2 negativity, grade 3 tumours, larger average tumour size and the shortest median time to disease progression and mortality. Despite these differences, the ABCFS, HEBCS and POSH stage-1 cohorts were similar in terms of the incidence of disease progression and mortality. In comparison, SUCCESS-A and POSH stage-2 had lower incidences of progression and mortality. To address these differences, survival analyses at stages 1 and 2 were adjusted for ER status and, for replicating SNPs, multivariable models were constructed using pooled data from stages 1 and 2.Stage-1 survival analysis and meta-analysis
Following standard quality control (QC, see Methods), 4739 patients from four cohorts ABCFS (N = 202), HEBCS (N = 798), POSH (N = 556) and SUCCESS-A (N = 3183) and 5,848,861 SNPs (813,964 observed and 5,034,897 imputed) were used for genome-wide analysis of overall survival (OS) at stage-1 (Table 1 and Supplementary Table 1). The ABCFS cohort was excluded from the early-onset and disease-free survival (DFS) analyses because data on progression were unavailable. Consequently, 4537 patients were used for DFS in all cases and 1102 patients for early-onset analyses at stage-1. Among the survival analyses performed at stage-1 there were 823 events for OS, 991 events for DFS (all cases), 326 events for early-onset OS and 391 events for early-onset DFS. According to these sample sizes and event rates, we estimated that the combined stage-1 analysis of OS and DFS had 80% power to detect common SNPs (minor allele frequency (MAF)=0.3) with modest effects (HR=1.4, P α = 5 × 10−8). Due to its smaller sample size, the analysis of OS and DFS in early-onset cases was estimated to have 80% power to detect SNPs with slightly larger effect sizes (OS: HR=1.7, DFS: HR=1.6, Supplementary Fig. 2).For each cohort, the quantile–quantile (QQ) plots and low genomic inflation factors (λ ≤ 1.05) for OS and DFS in all cases and in the early-onset subset demonstrate good agreement between observed and expected P-values until the tail of the distributions where SNPs with P-values <10−4 deviated from the null distribution (Supplementary Figs. 3–5). Systematic biases such as population stratification are therefore unlikely to contribute to the significance of these SNPs. Comparison of the QQ plots for OS and DFS showed that analyses of DFS tended to identify more SNPs with low P-values (P Cox regression ≤ 10−4) which is consistent with the larger number of events.
Following Cox regression, we used a fixed effects meta-analysis to combine evidence across the stage-1 cohorts and visualized these results in a Manhattan plot by selecting the most significant P-value for each SNP from the analysis of OS or DFS, in all cases and the early-onset subset (Fig. 1). Despite meta-analysis of upto 4739 patients and sufficient power to detect common SNPs (MAF=0.3) with modest effect sizes (HR=1.4 for all patients and HR=1.6 for early onset), no associations achieved genome-wide significance at stage-1. We therefore used our selection criteria (see Methods) to select 87 SNPs for assessment at stage-2, with P-values from the stage-1 meta-analysis ranging from 3.5 × 10−7 to 0.008 (Supplementary Data 1).
Genome-wide
analysis of breast cancer survival. The Manhattan plot shows the result
of the stage-1 meta-analysis. Results are plotted as –log10 of the P-value from Cox regression. For each SNP the most significant P-value
is selected from the analysis of either overall survival (OS) or
disease-free survival (DFS) in all patients or the subset with early
onset. The four most significant SNPs after meta-analysis of stages 1
and 2 are highlighted in green (rs410155 and rs12302097 associated with
OS and DFS respectively in the whole cohort and rs715212 and rs10963755
associated with DFS in patients with early onset). This plot was
produced using the qqman R package
Replication and meta-analysis of stages 1 and 2
For replication at stage-2, 83 of the 95 selected SNPs were successfully genotyped by LGC Genomics using KASP chemistry in 1303 patients from the POSH cohort. QC excluded two SNPs with significant deviation from Hardy–Weinberg equilibrium (P Hardy–Weinberg ≤ 1 × 10−10) and five monomorphic SNPs leaving 76 SNPs for analysis (Supplementary Data 1). After testing for association with OS and DFS, we identified three independent signals that were represented by four SNPs (1: rs715212 and rs10963755; 2: rs12302097; and 3: rs410155) with replication P-values from Cox regression ranging from 0.009 to 0.043 and effects in the same direction as the complimentary stage-1 analysis (Supplementary Data 1). For these SNPs, we used a fixed effects meta-analysis to determine their final effect size and significance by combining evidence from stages 1 and 2. Although none of the four SNPs reached a genome-wide level of significance, they were highly significant (P meta ranging from 3.54 × 10−5 to 1.28 × 10−4) and there was no evidence of heterogeneity between cohorts despite the reported differences in clinicopathologic features (Table 2). Furthermore, the association with rs715212 was determined to be noteworthy by the FPRP ≤ 0.2.
Forest
plot and meta-analysis for the four most significant SNPs associated
with overall survival (OS) or disease-free survival (DFS). Forest plot
showing the event rate, hazard ratio (HR), 95% confidence interval (CI)
and significance level (P-value) from Cox regression in each
cohort and the combined analysis for the most significant SNPs
associated with DFS and OS. ABCFS*: evidence for association with OS in
the ABCFS cohort is shown for each SNP but these results are excluded
from the meta-analyses of DFS. The SNP subtotal rows show the result for
a fixed effects meta-analysis across four studies for rs715212,
rs10963755 and rs12302097 and five studies for rs410155 using I
2 and Cochran Q-statistic to assess heterogeneity in effect sizes between cohorts
Although rs715212 and rs10963755 are only separated by 4.7 kb, the linkage disequilibrium between them is weak (r 2 = 0.33) and they were consequently treated as independent loci when selecting SNPs for replication. However, a multivariable analysis of DFS using pooled data in early-onset patients from HEBCS, POSH and SUCCESS-A showed that these SNPs represent a single association signal since rs10963755 did not retain significance (P pooled = 0.402) after adjusting for rs715212 (P pooled = 0.003).
rs12302097 is associated with an increased risk of relapse in all patients (P meta = 7.54 × 10−5, HR=1.30) with a similar effect in patients with early (P meta = 6.77 × 10−5, HR=1.45) and late onset (P meta = 0.0742, HR=1.19, Table 2). Finally, rs410155 is associated with an increased risk of mortality in all cases (P meta = 1.28 × 10−4, HR=1.34), with similar effect in patients with early (P meta = 0.0049, HR=1.32) and late onset (P meta = 0.0066, HR=1.32). Results from all survival and meta-analyses for the 95 SNPs that were selected for genotyping at stage-2 are given in Supplementary Data 1.
Univariate Kaplan–Meier (KM) plots for the replicating SNPs and most significant outcomes were produced using pooled data from stages 1 and 2 (Fig. 3). Excluding rs410155, the significance levels and effect sizes from the pooled analyses were very similar to those obtained from meta-analysis (rs715212 P pooled = 1.94 × 10−5, HR=1.28; rs10963755 P pooled = 6.48 × 10−4, HR=1.21; rs12302097 P pooled = 1.65 × 10−4, HR=1.28, Table 3). For rs410155, the pooled analysis was less significant and the HR was slightly smaller (P pooled = 0.015, HR=1.20). The KM plots for rs715212 and rs12302097 were consistent with the additive model tested while the plots for rs10963755 and rs410155 were indicative of a dominant effect. For rs10963755 and rs410155, the dominant model was equally significant and the effect sizes were slightly larger (rs10963755 P dominant = 6.46 × 10−4, HR=1.30; rs410155 P dominant = 0.0120, HR=1.23).
Kaplan–Meir
survival plots for the four most significant SNPs identified by
meta-analyses. Kaplan–Meier plots from univariate analysis of the most
significant SNP associated with disease-free survival (DFS) in cases
with early onset (a, rs715212 and b, rs10963755), DFS in all cases (c, rs12302097) and overall survival (OS) in all cases (d,
rs410155). For OS, the data from all five cohorts (ABCFS, HEBCS, POSH
stages 1 and 2 and SUCCESS-A) was pooled whereas for DFS data were
pooled across four cohorts because DFS was not recorded in the ABCFS
cohort. HR: hazard ratio with 95% confidence interval
Multivariable models
We used multivariable Cox regression in pooled data from stages 1 and 2 to determine whether the replicating SNPs were independent of the known prognostic factors that were available across all studies except ABCFS. After adjustment for ER status, tumour grade, maximum tumour diameter, axillary nodal status and study, the association between rs715212 and risk of disease progression in patients with early onset approached a genome-wide level of significance (P multivariable = 5.37 × 10−8, HR=1.38, Table 3). The associations between rs10963755 and DFS in patients with early onset (P multivariable = 4.51 × 10−5, HR=1.27) and between rs410155 and OS in all cases (P multivariable = 0.0023, HR=1.28), also became stronger after adjustment for tumour characteristics and study compared with univariate analysis. For rs12302097, the association with DFS in all cases (P multivariable = 0.001, HR=1.26) was less significant after adjustment.Functional inference
To explore the functional relevance of the regions associated with survival, we used HaploReg v4.121, RegulomeDB22 and SeattleSeq23 to determine if the risk SNPs and their proxies (r 2 ≥ 0.2) are located within putative functional elements such as active histone marks or transcription factor (TF) binding motifs (Supplementary Data 3). rs715212 and rs10963755 are located in intron 19 of the ADAMTSL1 gene and both are reported to alter TF binding motifs (Fig. 4a and Supplementary Data 3). The chromatin surrounding rs715212 is characterized as an enhancer in breast myoepithelial primary cells (strongly enriched for TF binding sites, moderately enriched for DNase peaks and conserved elements), while rs10963755 maps to a quiescent region. rs12302097 is located in a 64 kb region of linkage disequilibrium (LD) (r 2 > 0.2) between carbohydrate sulfotransferase 11 (CHST11, 38 kb upstream) and thioredoxin reductase 1 (TXNRD1, 68.5 kb downstream). The chromatin in this region has properties of weak transcription in variant human mammary epithelial cells (vHMECs) and the SNP has been shown to alter several TF binding motifs (Fig. 4b and Supplementary Data 3). Finally, rs410155 is located between two metallothionein genes: MT3 (8.7 kb upstream) and MT4 (11.6 kb downstream, Fig. 4c). Although the surrounding chromatin in breast myoepithelial primary cells is quiescent, this SNP has also been predicted to alter TF binding motifs.
Regional
plots of association with survival (OS or DFS) at stage-1
meta-analysis, recombination rate and gene context for the most
significant SNPs. Results from the stage-1 meta-analyses in a region
surrounding the most significant SNP associated with DFS in patients
with early onset (a, rs715212 and rs10963755), DFS in all patients (b, rs12302097) and OS in all patients (c,
rs410155). In each plot, a purple diamond identifies the index SNP and
the colour of other SNPs represent their linkage disequilibrium (r
2) with the index SNP from light blue (r
2 ≤ 0.4) to red (r
2 ≥ 0.8). The middle
panel displays the 15 state chromatin segmentation track (ChromHMM) in
breast variant human mammary epithelial cells (vHMECs, E028), mammary
epithelial primary cells (HMECs, E119) and breast myoepithelial primary
cells (E027) using data from the HapMap ENCODE Project. The lower panels
show genes and their direction of transcription (arrows). Physical
positions are relative to build 37 (hg19) of the human genome
Several genes related to ADAMTSL1 have been implicated in the development of normal breast tissue and in the initiation and progression of breast cancer including ADAMTS126, ADAM1027, ADAM1228 and ADAM1729. Of these, ADAM17, which plays a key role in normal breast development via its cleavage and release of amphiregulin (AREG) from the surface of breast epithelial cells, appeared to be particularly relevant because two independent studies have shown that AREG is overexpressed in ER-positive breast tumours from premenopausal women vs. postmenopausal women17,18. We therefore used GTEx to perform further eQTL analysis, and found that rs715212 is nominally associated with the expression of AREG (P FastQTL = 0.035) in breast mammary tissue (Supplementary Fig. 6).
Triple-negative breast cancer
TNBC is an aggressive breast cancer subtype with limited treatment options due to the lack of expression of ER, PR and HER2 receptors. Studies have shown that the immunoreactivity of MT3, which flanks rs410155, is associated with poor prognosis in TNBC patients30,31. To explore the relationship with TNBC, the survival analyses were repeated in a pooled subset of TNBC patients. rs410155 was the only SNP that had a stronger association with prognosis in patients with TNBC (P pooled = 0.004 for OS in TNBC vs. P pooled = 0.014 for OS in all cases, Supplementary Table 3 and Supplementary Fig. 7).Discussion
A
recent study highlighted the difficulties of detecting variants linked
to breast cancer survival that were predicted to have small effect sizes10.
They suggested that very large sample sizes will be required to detect
common variants conferring HRs that may be as low as 1.1, which is in
line with findings from GWASs of breast cancer risk. To our knowledge,
only two loci, rs445820432 and rs205961433,
have confidently been associated with breast cancer survival at
genome-wide levels of significance despite the analysis of up to 37,954
patients and an event rate of 7.6% (n = 2900 deaths). Our
hypothesis was that enrichment for well-characterized early-onset cases
with worse prognosis might facilitate detection by increasing the event
rate, reducing genetic heterogeneity and because variants with larger
effect sizes might underlie more aggressive forms of disease. Although
the present study has a comparatively small sample size (n = 6042
patients including 2315 aged ≤ 40 years at diagnosis), the event rate
is significantly higher over all patients (OS 18.2%, DFS 22.5%) and the
early-onset subset (OS 26.1%, DFS 30.9%) which is one of the main
determinants of power. Consequently, the combined analysis of stages 1
and 2 for DFS is estimated to have 80% power to detect common SNPs
(MAF=0.3) with an effect size of HR=1.31 in all patients and HR=1.42 in
the early-onset subset at a genome-wide level of significance.
Despite sufficient statistical power to detect common SNPs with modest effect sizes no variants were identified at a genome-wide level of significance. This may reflect the confounding impacts of a large number of factors that influence survival times, including phenotypic heterogeneity, tumour biology and treatment. However, three signals had suggestive levels of significance (1: rs715212 and rs10963755; 2: rs12302097; and 3: rs410155) without heterogeneity between cohorts including one that was estimated to be noteworthy according to the FPRP (rs715212 FPRP< 0.2). With the exception of rs12302097, the effect size (HR) and significance of these associations became stronger after adjustment for tumour characteristics in multivariable models. This suggests that tumour characteristics are confounding factors and that accounting for them in a multivariable model will increase the accuracy of effect size estimates. Previous studies have shown that similar adjustments for known prognostic factors increases statistical power in the analysis of time-to-event outcomes34.
The signal involving rs715212 and rs10963755 was associated exclusively with disease progression in patients with early onset and, in a pooled analysis with adjustment for tumour characteristics and study, rs715212 approached a genome-wide level of significance. However, for both SNPs there was no difference in risk allele frequency between patients with early and late onset. This suggests that the association may involve an interaction with another factor that is unique to early-onset patients such as the expression or somatic mutation profile of the tumour.
Both rs715212 and rs10963755 are located in intron 19 of the ADAMTSL1 gene which encodes a secreted glycoprotein and is a member of the ADAMTS (a disintegrin and metalloproteinase with thrombospondin motif) family (Fig. 4a). Previous studies have shown that ADAMTSL1 is a component of the extracellular matrix that may function in cell–cell or cell–matrix interactions or may regulate other ADAMTS proteases35. Although ADAMTSL1 is primarily expressed in skeletal muscle, it has been seen in other tissues including breast and methylation studies have shown that it is hypermethylated in ER-positive breast cancer tumours36,37.
We have shown that rs715212 is nominally associated with the expression of AREG and that the chromatin surrounding rs715212 is predicted to be functional. These findings provide tentative evidence that rs715212 and/or linked variants may influence disease progression in early-onset patients by altering the methylation or functionality of ADAMTSL1 and/or the expression of AREG either directly or via regulation of other members of the ADAMTS gene family. However, it is important to stress that further functional studies are required to verify the association between rs715212 and AREG expression and determine the biological mechanism.
Of the two remaining SNPs with moderate association, rs12302097 is associated with disease progression in all patients and rs410155 is associated with OS in all patients. For rs12302097, we have shown that SNPs in weak LD are associated with the expression of both flanking genes (CHST11 and TXNRD1) and both of these genes have previously been associated with breast cancer38,39,40,41. This suggests that CHST11 and or TXNRD1 may influence prognosis.
The final variant, rs410155, is located between two metallothionein genes, MT3 and MT4. While MT4 has not been associated with breast cancer, MT3 is overexpressed in breast cancer cells, which is associated with increased invasiveness and higher concentrations of matrix metallopeptidase 330,31. These findings suggest that elevated expression of MT3 may underlie the association that we have identified between overall survival and genetic variation at rs410155.
The genome-wide significant SNPs identified by previous studies were both associated with survival in ER-negative patients32,33. We therefore repeated the stage-1 meta-analysis using ER-negative patients only (n = 1637) but failed to replicate the findings for rs4458204 (P meta = 0.771) and rs2059614 (P meta = 0.482) despite a small overlap in the patients tested by these studies (n = 196 from HEBCS for rs2059614 and n = 315 from POSH for rs4458204). However, the current study lacks power to replicate these findings given the small number of ER-negative patients and low MAF for rs4458204 (MAF=0.12) and rs2059614 (MAF=0.03).
In a subsequent study which aimed to replicate SNPs with suggestive significance levels using the same patient cohorts, Pirie et al.10 identified 12 variants with nominal significance (P < 0.05) including 7 that were associated with ER-positive disease. Eleven of these SNPs were genotyped and tested by the current study which replicated the association with rs1800566 in all patients (P meta = 0.01) and rs10477313 in ER-positive patients (P meta = 0.013). The nine remaining variants showed no evidence of association, although five of these had MAF < 0.1.
The current study has several limitations which must be noted. First, no variants were identified at a genome-wide level of significance and the most significant results were derived from a multivariable analysis which adjusted for the confounding effect of tumour characteristics. Second, none of the survival analyses were adjusted for treatment and the ABCFS cohort could not be included in the analyses of DFS, early onset and multivariable models because these variables were unavailable. Third, although the study is well powered to detect common SNPs we estimate that it only has 20% power to detect rare SNPs (MAF=0.1) associated with DFS with HRs of 1.31 for all patients and 1.44 for those with early onset. Fourth, the associations with gene expression involve healthy participants and have relatively modest significance levels. Further analysis with larger sample sizes and adjustment for additional clinicopathological factors including treatment (chemotherapy and hormone therapy) may provide more information that could further improve survival analysis. Further functional studies involving breast cancer patients and including epigenetic mechanisms should be performed to provide more insights about the three association signals identified in the present study.
Our meta-analysis identified three independent signals associated with breast cancer prognosis that are independent of the classical prognostic factors. Interestingly, the signal located in ADAMTSL1 was only associated with disease progression in patients with early onset. This suggests that unique disease mechanisms may influence survival in younger women and provide some biological insight into why younger-onset breast cancer has a worse prognosis. We have also discussed the possible impact of these variants on the methylation of ADAMTSL1, its interaction with other members of the ADAMTS gene family and their association with the expression of other biologically relevant genes such as AREG in the context of breast cancer prognosis. The SNPs identified in this study have the potential to improve the accuracy of prognostic estimates and stratification of patients into treatment groups. Moreover, the gene implicated by these SNPs may warrant further investigation as novel therapeutic targets and some are already under investigation for this purpose.
Despite sufficient statistical power to detect common SNPs with modest effect sizes no variants were identified at a genome-wide level of significance. This may reflect the confounding impacts of a large number of factors that influence survival times, including phenotypic heterogeneity, tumour biology and treatment. However, three signals had suggestive levels of significance (1: rs715212 and rs10963755; 2: rs12302097; and 3: rs410155) without heterogeneity between cohorts including one that was estimated to be noteworthy according to the FPRP (rs715212 FPRP< 0.2). With the exception of rs12302097, the effect size (HR) and significance of these associations became stronger after adjustment for tumour characteristics in multivariable models. This suggests that tumour characteristics are confounding factors and that accounting for them in a multivariable model will increase the accuracy of effect size estimates. Previous studies have shown that similar adjustments for known prognostic factors increases statistical power in the analysis of time-to-event outcomes34.
The signal involving rs715212 and rs10963755 was associated exclusively with disease progression in patients with early onset and, in a pooled analysis with adjustment for tumour characteristics and study, rs715212 approached a genome-wide level of significance. However, for both SNPs there was no difference in risk allele frequency between patients with early and late onset. This suggests that the association may involve an interaction with another factor that is unique to early-onset patients such as the expression or somatic mutation profile of the tumour.
Both rs715212 and rs10963755 are located in intron 19 of the ADAMTSL1 gene which encodes a secreted glycoprotein and is a member of the ADAMTS (a disintegrin and metalloproteinase with thrombospondin motif) family (Fig. 4a). Previous studies have shown that ADAMTSL1 is a component of the extracellular matrix that may function in cell–cell or cell–matrix interactions or may regulate other ADAMTS proteases35. Although ADAMTSL1 is primarily expressed in skeletal muscle, it has been seen in other tissues including breast and methylation studies have shown that it is hypermethylated in ER-positive breast cancer tumours36,37.
We have shown that rs715212 is nominally associated with the expression of AREG and that the chromatin surrounding rs715212 is predicted to be functional. These findings provide tentative evidence that rs715212 and/or linked variants may influence disease progression in early-onset patients by altering the methylation or functionality of ADAMTSL1 and/or the expression of AREG either directly or via regulation of other members of the ADAMTS gene family. However, it is important to stress that further functional studies are required to verify the association between rs715212 and AREG expression and determine the biological mechanism.
Of the two remaining SNPs with moderate association, rs12302097 is associated with disease progression in all patients and rs410155 is associated with OS in all patients. For rs12302097, we have shown that SNPs in weak LD are associated with the expression of both flanking genes (CHST11 and TXNRD1) and both of these genes have previously been associated with breast cancer38,39,40,41. This suggests that CHST11 and or TXNRD1 may influence prognosis.
The final variant, rs410155, is located between two metallothionein genes, MT3 and MT4. While MT4 has not been associated with breast cancer, MT3 is overexpressed in breast cancer cells, which is associated with increased invasiveness and higher concentrations of matrix metallopeptidase 330,31. These findings suggest that elevated expression of MT3 may underlie the association that we have identified between overall survival and genetic variation at rs410155.
The genome-wide significant SNPs identified by previous studies were both associated with survival in ER-negative patients32,33. We therefore repeated the stage-1 meta-analysis using ER-negative patients only (n = 1637) but failed to replicate the findings for rs4458204 (P meta = 0.771) and rs2059614 (P meta = 0.482) despite a small overlap in the patients tested by these studies (n = 196 from HEBCS for rs2059614 and n = 315 from POSH for rs4458204). However, the current study lacks power to replicate these findings given the small number of ER-negative patients and low MAF for rs4458204 (MAF=0.12) and rs2059614 (MAF=0.03).
In a subsequent study which aimed to replicate SNPs with suggestive significance levels using the same patient cohorts, Pirie et al.10 identified 12 variants with nominal significance (P < 0.05) including 7 that were associated with ER-positive disease. Eleven of these SNPs were genotyped and tested by the current study which replicated the association with rs1800566 in all patients (P meta = 0.01) and rs10477313 in ER-positive patients (P meta = 0.013). The nine remaining variants showed no evidence of association, although five of these had MAF < 0.1.
The current study has several limitations which must be noted. First, no variants were identified at a genome-wide level of significance and the most significant results were derived from a multivariable analysis which adjusted for the confounding effect of tumour characteristics. Second, none of the survival analyses were adjusted for treatment and the ABCFS cohort could not be included in the analyses of DFS, early onset and multivariable models because these variables were unavailable. Third, although the study is well powered to detect common SNPs we estimate that it only has 20% power to detect rare SNPs (MAF=0.1) associated with DFS with HRs of 1.31 for all patients and 1.44 for those with early onset. Fourth, the associations with gene expression involve healthy participants and have relatively modest significance levels. Further analysis with larger sample sizes and adjustment for additional clinicopathological factors including treatment (chemotherapy and hormone therapy) may provide more information that could further improve survival analysis. Further functional studies involving breast cancer patients and including epigenetic mechanisms should be performed to provide more insights about the three association signals identified in the present study.
Our meta-analysis identified three independent signals associated with breast cancer prognosis that are independent of the classical prognostic factors. Interestingly, the signal located in ADAMTSL1 was only associated with disease progression in patients with early onset. This suggests that unique disease mechanisms may influence survival in younger women and provide some biological insight into why younger-onset breast cancer has a worse prognosis. We have also discussed the possible impact of these variants on the methylation of ADAMTSL1, its interaction with other members of the ADAMTS gene family and their association with the expression of other biologically relevant genes such as AREG in the context of breast cancer prognosis. The SNPs identified in this study have the potential to improve the accuracy of prognostic estimates and stratification of patients into treatment groups. Moreover, the gene implicated by these SNPs may warrant further investigation as novel therapeutic targets and some are already under investigation for this purpose.
Methods
Patient cohorts for discovery and replication stages
At stage-1, breast cancer samples were selected from four cohorts: (1) ABCFS (http://epi.unimelb.edu.au/research/cancer/breast/)42, (2) HEBCS43, (3) POSH from the United Kingdom44 and (4) a prospective randomized phase III clinical trial comparing FEC-Doc Chemotherapy vs. FEC DocG chemotherapy (SUCCESS-A) from Germany45. Prior to QC, these data sets consisted of: 214 incident cases in ABCFS diagnosed between 1992 and 1995 with a first primary invasive breast cancer before the age of 40 years and living in Melbourne or Sydney; 832 breast cancer patients in HEBCS aged 22–96 years and treated in the Helsinki region between 1997 and 2004; 574 patients from the POSH study aged ≤ 40 years at diagnosis of invasive breast cancer between 2000 and 2007; and 3277 patients from the SUCCESS-A trial diagnosed with primary epithelial invasive carcinoma and recruited between 2005 and 2007 (Supplementary Table 1). All patients gave informed consent and the studies were approved by the relevant ethics committees42,43,44,45. Histopathological and survival data were collected for all patients. In HEBCS, 590 patients were unselected and 242 were familial43. In the POSH cohort, 274 patients were genotyped as part of a larger study on TNBC in which there is little or no tumour expression for ER, PR and human epidermal growth factor receptor 2 (HER2)46. To increase power, the remaining samples from the POSH cohort were enriched for survival extremes corresponding to patients with early distant metastasis or death (n = 193, median OS=2.8 years) and patients with long-term event free survival (n = 96, median OS=8.7 years).A further 1303 patients from the POSH cohort44 who were unselected for any survival differences and were independent of the stage-1 data set were used for replication analysis at stage-2 (Supplementary Table 1). These patients were aged ≤ 40 years at diagnosis of invasive breast cancer and had self-reported ethnicities of White/Caucasian (n = 1285), eastern European (n = 3), Greek (n = 2), South African (n = 2) and Jewish (n = 11).
To assess the similarity of clinicopathologic features between all cohorts with data (n = 3 to 5), Pearson’s χ2 test was used for categorical traits and Kruskal–Wallis rank sum tests were used for continuous traits (Table 1).
Genotyping and QC at stage-1
At stage-1, samples were genotyped using the Illumina 610k array for ABCFS, Illumina 550K array for HEBCS47, Illumina 660-Quad array for POSH by The Mayo Clinic (Rochester, MN, USA) and Genome Institute of Singapore (GIS)48 and the HumanOmniExpress-FFPE BeadChip for SUCCESS-A. Standard QC measures were applied to the genotypic data from stage-1, which removed SNPs with MAFs of < 5%, SNPs and individuals with > 10% missing genotypes and SNPs with significant deviations from Hardy–Weinberg equilibrium (P-value ≤ 1 × 10−10). Strand issues, where the allele coding differs between cohorts, were resolved by combining the cohorts and flipping strands for SNPs with more than two alleles and by ensuring that the MAFs were similar between cohorts particularly for A/T and G/C SNPs where strand issues cannot be detected by allelic excess. The reported gender of the individuals was verified against that predicted from their genotypic data. All duplicate samples, individuals with incomplete phenotypic data for survival analyses and samples that were cryptically related (pairwise-identity by state > 86%) were excluded from the stage-1 cohorts. Samples that were inferred to have non-European ancestry by multidimensional scaling analysis against reference populations from HapMap were excluded so that the remaining samples in each cohort formed a single cluster which overlapped with the Caucasian reference (CEU, Supplementary Fig. 1). Across the four stage-1 cohorts, a total of 143,380 SNPs and 158 patients were removed during these QC steps leaving 4739 patients and observed genotypes at 813,964 SNPs for analysis. The number of SNPs and patients removed and remaining in each cohort is shown in Supplementary Table 1. All QC procedures were carried out using PLINK49.Imputation of the stage-1 data and further QC
To aid meta-analysis and to increase the resolution of the stage-1 data, additional SNPs were imputed using MACH 1.0 (http://www.sph.umich.edu/csg/abecasis/MACH/index.html). The reference data for imputation were SNP genotypes from HapMap phase 2 and phased haplotypes from the CEPH population (Utah residents with ancestry from northern and western Europe, CEU). The imputed genotypes were quality controlled by excluding SNPs with: a posterior probability < 0.9, MAF <5%, >10% missing genotypes or significant deviations from Hardy–Weinberg equilibrium (P-value ≤ 1 × 10−10). Following these QC steps, 5,848,861 imputed SNPs across the four stage-1 cohorts remained for analysis (Supplementary Table 1).Power calculations
The power to detect SNPs associated with OS and DFS in all cases and patients with early-onset breast cancer in the combined stage-1 cohorts was estimated using the survSNP program in R50 with an additive genetic risk model and type 1 error rate (α) of 5 × 10−8 (Supplementary Fig. 2). A range of modest genotype HRs (1.1–2.0) and risk allele frequencies (0.05–0.3) were used along with the documented values for sample size and event rate after QC (Supplementary Table 1).Cox regression
To identify SNPs influencing prognosis we used the formetascore command in GenABEL51 to perform Cox regression analyses of OS and DFS, with correction for ER status which is the only variable that is recorded in all four cohorts, has the most complete data and is associated with survival52. SNPs were coded according to the number of rare alleles (0–2). For OS, follow-up times were defined as the duration between breast cancer diagnosis and death from any cause or last follow-up if alive. For DFS, the follow-up times were defined as the time between diagnosis and disease progression in the form of local recurrence, distant metastasis or death from any cause, whichever occurred first, or last follow-up if alive. Patients who had not experienced an event at the time of analysis were censored at their date of last follow-up.To identify variants associated with early-onset breast cancer, the OS and DFS analyses were repeated in a subset of 2315 patients who were aged ≤ 40 years at diagnosis from HEBCS (n = 119), POSH stage-1 (n = 556), POSH stage-2 (n = 1303) and SUCCESS-A (n = 337). For the early-onset analysis, the POSH cohort was particularly important because all of the POSH patients were aged ≤ 40 years at diagnosis. The ABCFS data set did not contain data on age of onset, local recurrence or distant metastasis and therefore could not be used for the analysis of DFS or early onset. For SNPs associated with early onset, the relationship between prognosis and onset age was further explored by: (1) repeating the OS and DFS survival analyses in a subset of patients from HEBCS (n = 679) and SUCCESS-A (n = 2846) that were aged > 40 years at diagnosis and (2) testing for allelic association with OS and DFS events in patients with early (aged ≤40 years at diagnosis) and late onset (aged > 40 years at diagnosis) using Pearson's χ2 test.
The mean difference in time between age at diagnosis and age at registration was 0.78 years (s.d.=1.16 years) over all cohorts.
Visualization of stage-1 results
To verify the robustness of our QC measures and to examine the possibility of confounding factors such as population stratification, we used the qqnorm and qqplot procedures in R53 to generate QQ plots of the observed and expected P-values under the null distribution in the stage-1 data (Supplementary Figs. 3–5).To visualise the stage-1 results and SNPs selected for follow-up, the qqman package in R54 was used to generate a Manhattan plot and highlight the most significant SNPs associated with OS and DFS (Fig. 1). For SNPs with significant replication, Locus Zoom55 was used to generate regional plots of the stage-1 data to show the pattern of association surrounding the index SNP with respect to LD with neighbouring SNPs, underlying recombination rate and gene context (Fig. 4).
Meta-analysis
To select SNPs for follow-up at stage-2, we used PLINK49 to perform a fixed effects inverse variance-weighted meta-analysis of the stage-1 results for OS and DFS in all cases and the subset of patients with early onset. A fixed effects meta-analysis was used under the assumption that SNPs have one true effect size and that any differences between studies were most likely to be due to sampling variation. To estimate heterogeneity in effect size between studies we used the χ 2-based Cochran Q-statistic and I 2 which gives the percentage of variation across studies that is due to heterogeneity rather than chance56. The same methodology was used to determine the final significance and effect size of SNPs by meta-analysis of the Cox regression results from stages 1 and 2. To visualise the meta-analysis, we produced a forest plot using Stata version 12 (Fig. 2)57. Following meta-analysis of stages 1 and 2, data from all cohorts were pooled and KM plots were generated for the most significant SNPs using Stata version 1257.False positive report probability
To assess the reliability of the associations from meta-analysis of stages 1 and 2 we calculated the FPRP which describes the probability of no true association between a genetic variant and disease, given a statistically significant finding58. The FPRP was calculated using a low prior probability of 0.0001, which is expected for a random SNP, to detect a hazard ratio of 1.3. A threshold of FPRP ≤ 0.2 was used to identify noteworthy associations.Selection, genotyping and QC of SNPs at stage-2
Completely unbiased methods of SNP selection have no means of excluding false positives which are likely to be among the most significant signals. They will also neglect moderately significant SNPs in favour of the most significant SNPs despite potentially overwhelming support from correlated SNPs and proximity to biologically relevant genes. To select the most promising SNPs for follow-up, we therefore used a clumping procedure in PLINK49 to generate a shortlist of index SNPs with support from correlated SNPs (SNPs r 2 ≥ 0.5, within 500 kb). Priority, but not exclusivity, was then given to index SNPs that were close to a relevant gene according to annotation from GeneAlacart (https://genealacart.genecards.org/). Two shortlists of index SNPs were made which used either a stringent (index SNP P meta ≤ 0.001 and correlated SNP P meta ≤ 0.01) or moderate set of P-value thresholds (index SNP P meta ≤ 0.01 and correlated SNP P meta ≤ 0.1). SNPs were selected from the stringent shortlist first (n = 50) and then from the moderate shortlist (n = 37). Since priority but not exclusivity was given to SNPs close to relevant genes, 20 SNPs were selected on a completely unbiased basis and 67 were selected from the unbiased shortlist because they were close to a relevant gene (Supplementary Data 1).To select additional SNPs, we performed a literature search, which identified 73 variants that have previously been associated with breast cancer survival (OS, DFS or breast cancer-specific survival) in independent cohorts. These published SNPs were cross-referenced with our stage-1 meta-analysis and 8 additional SNPs were selected on the basis of their published association with onset age and/or because the gene implicated had potential applications for diagnosis, risk prediction or therapeutic intervention.
The 95 SNPs selected for replication were genotyped by LGC Genomics (Hoddeson, UK) in 1303 patients from the POSH cohort. The genotypes were quality controlled by excluding SNPs with >10% duplicate error rate, >10% missing genotypes or significant deviations from Hardy–Weinberg equilibrium (P-value ≤ 1 × 10−10).
Multivariable Cox regression
We used multivariable Cox regression in pooled data from stages 1 and 2 to determine whether SNPs with the most significant impacts on survival were independent of the known prognostic factors that were available across all studies. Data on ER status (negative=0, positive=1) tumour grade (1 to 3), maximum tumour diameter (mean 25.7, range 0 to 220 mm) and axillary nodal status (not affected=0, affected=1) were available for 93% of the cases that passed QC (n = 5622/6042). These prognostic factors along with the cohort used were treated as covariates and were entered into the proportional hazards model in order of significance. All survival analyses were carried out using Stata version 12 and the P-values reported were two-sided at 5% significance.Functional annotation of significant SNPs
To explore the functional relevance of the regions associated with survival, we used HaploReg v4.121, RegulomeDB22 and SeattleSeq23 to interrogate ENCODE data59 and annotate the risk SNPs and their linked SNPs (r 2 ≥ 0.2) with respect to: histone modifications, DNAseI hypersensitivity, proteins bound, disruption of regulatory motifs, conservation metrics from genomic evolutionary rate profiling (GERP)60 and combined annotation-dependent depletion scores (CADD)61, and functionality scores from RegulomeDB. The scores from RegulomeDB were generated using data from Gene Expression Omnibus (GEO), ENCODE and published literature. Variants with a RegulomeDB score of 3 are likely to affect binding while variants scoring 4–6 have minimal evidence for functional activity.Additionally, candidate regions were annotated with 15 state chromatin segmentation in breast vHMECs (E028), mammary epithelial primary cells (HMEC, E119) and breast myoepithelial primary cells (E027). These chromatin states categorize noncoding DNA into functional regulatory elements such as enhancers and quiescent regions that are respectively enriched and depleted for phenotype-associated SNPs62. The chromatin states were generated by computational integration of binarized chromatin immunoprecipitation sequencing data using a multivariable Hhidden Markov model that explicitly models the combinatorial patterns of observed modifications63.
To gain further functional insight, eQTL analysis was performed for all SNPs in LD (r 2 ≥ 0.2) with the index SNPs using the GTEx portal (V6, dbGaP Accession phs000424.v6.p1)24 to query RNAseq data from breast mammary tissue in 183 samples with genotype data.
Data availability
All relevant summary statistics from the ABCFS, HEBCS and POSH cohorts are available from the authors for collaborative research upon request to the corresponding author. The SUCCESS-A data are available via authorized access from dbGaP (Study Accession: phs000547.v1.p1).
Subscribe to:
Posts (Atom)